Christians Shouldn’t Be Dismissive of Scientific Modeling
Image
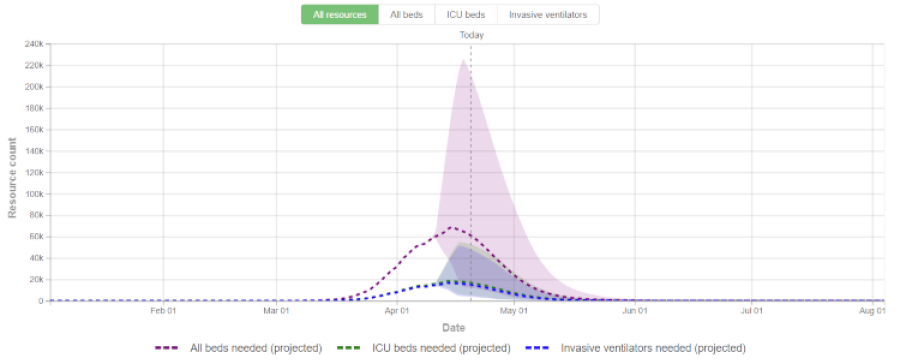
Projections from an IHME model.
Over the last several weeks I’ve encountered a range of negative views toward the models epidemiologists have been using in the struggle against COVID-19. Skepticism is a healthy thing. But rejecting models entirely isn’t skepticism. Latching onto fringe theories isn’t skepticism. Rejecting the flattening-the-curve strategy because it’s allegedly model-based isn’t skepticism either.
These responses are mostly misunderstandings of what models are and of how flattening-the-curve came to be.
I’m not claiming expertise in scientific modeling. Most of this is high school level science class stuff. But for a lot of us, high school science was a long time ago, or wasn’t very good—or we weren’t paying attention.
What do models really do?
Those tasked with explaining science to us non-scientists define and classify scientific models in a variety of ways.
The Stanford Encyclopedia of Philosophy, for example, describes at least 8 varieties of models, along with a good bit of historical and philosophical background. They’ve got about 18,000 words on it.
A much simpler summary comes from the Science Learning Hub, a Science-education project in New Zealand. Helpfully, SLH doesn’t assume readers have a lot of background.
In science, a model is a representation of an idea, an object or even a process or a system that is used to describe and explain phenomena that cannot be experienced directly. Models are central to what scientists do, both in their research as well as when communicating their explanations. (Scientific Modeling)
Noteworthy here: models are primarily descriptive, not predictive. Prediction based on a model is estimating how an observed pattern probably extends into what has not been observed, whether past, present, or future.
Encyclopedia Britannica classifies models as physical, conceptual, or mathematical. It’s the mathematical models that tend to stir up the most distrust and controversy, partly because the math is way beyond most of us. We don’t know what a “parametrized Gaussian error function” is (health service utilization forecasting team, p.4; see also Gaussian, Error and Complementary Error function).
But Christians should be the last people to categorically dismiss models. Any high school science teacher trained in a Christian university can tell you why. I’ve been reminded why most recently in books by Nancy Pearcy, Alvin Plantinga, William Lane Craig, and Samuel Gregg: Whether scientists acknowledge it or not, the work of science is only possible at all because God created an orderly world in which phenomena occur according to patterns in predictable ways. For Christians, scientific study—including the use of models to better understand the created order—is study of the glory of God through what He has made (Psalm 19:1).
Most of us aren’t scientists, but that’s no excuse for scoffing at one of the best tools we have for grasping the orderliness of creation.
Should we wreck our economy based on models?
The “models vs. the economy” take on our current situation doesn’t fit reality very well. Truth? The economy is also managed using models. A few examples:
- Calculating the unemployment rate
- Unemployment forecasting (also this)
- Business forecasting
- Cost Modeling
Beyond economics, modeling is used all the time for everything from air traffic predictions to vehicle fire research, to predictive policing (no, it isn’t like “Minority Report”).
Models are used extensively in all sorts of engineering. We probably don’t even get dressed in the morning without using products that are partly the result of modeling—even predictive modeling—in the design process.
Christians should view models as tools used by countless professionals—many of whom are believers—in order to try to make life better for people. Pastors have books and word processors. Plumbers have propane torches. Engineers and scientists have models. They’re all trying to help people and fulfill their vocations.
(An excellent use of predictive mathematical modeling…)
Why are models often “wrong”?
An aphorism about firearms says, “Guns don’t kill people; people kill people.” Implications aside, it’s a true statement. It’s also true that math is never wrong; people are wrong. Why? Math is just an aspect of reality. In response to mathematical reality, humans can misunderstand, miscalculate, and misuse, but reality continues to be what it is, regardless.
The fact that the area of a circle is always its radius squared times an irrational (unending) number we call “pi” (π) remains true, no matter how many times I misremember the formula, plug the wrong value in for π, botch the multiplication, or incorrectly measure the radius.
The point is that models, as complex representations of how variables relate to each other and to constants, are just math. In that sense, models are also never “wrong”—just badly executed or badly used by humans. That said, a model is usually developed for a particular purpose and can be useless or misleading for the intended purpose, so, in that sense, “wrong.”
When it comes to using models to find patterns and predict future events, much of the trouble comes from unrealistic expectations. It helps to keep these points in mind:
- Using models involves inductive reasoning: data from many individual observations is used in an effort to generalize.
- Inductive reasoning always results in probability, never certainty.
- The more data a model is fed, and the higher the quality of that data, the more probable its projections will be.
- When data is missing for parts of the model, assumptions have to be made.
- Changes in a model’s predictions are not really evidence of “failure.” As the quantity and quality of data changes, and assumptions are replaced with facts, good models change their predictions.
- True professionals, whether scientists or other kinds of analysts, know that models of complex data are only best guesses—and they don’t claim otherwise.
- The professionals that develop and use models in research are far more tentative and restrained in their conclusions than people who popularize the findings (e.g., the media).
In the case of COVID-19, one of the most influential models has been one of IHME’s (Institute for Health Metrics and Evaluation). Regarding that model, an excellent Kaiser Family Foundation article notes:
Models often present “best guess” or median forecasts/projections, along with a range of uncertainty. Sometimes, these uncertainty ranges can be very large. Looking at the IHME model again, on April 13, the model projected that there would be a 1,648 deaths from COVID-19 in the U.S. on April 20, but that the number of deaths could range from 362 to 4,989.
Poor design and misuse have done some damage to modeling’s reputation. Some famous global-warming scandals come to mind. But in the “Climategate” controversy, for example, raw data itself was apparently falsified. The infamous hockey stick graph appears to have involved both manipulated raw data and misrepresentation of what the model showed. Modeling itself was not the problem.
(XKD isn’t completely wrong … there is such a thing as “better garbage”)
Why bother with models?
Given the uncertainty built into predictive mathematical models, why bother to use them? Usually, the answer is “because we don’t have anything better.” Models are about providing decision-makers, who don’t have the luxury of waiting for certainty, with evidence so they don’t have to rely completely on gut instinct. It’s not evidence that stands alone. It’s not incontrovertible evidence. It’s an effort to use real-world data to detect patterns and anticipate what might happen next.
As for COVID-19, the idea that too many sick at once would overwhelm hospitals and ICUs, and that distancing can help slow the infection rate and avoid that disaster, isn’t a matter of inductive-reasoning from advanced statistical models. It’s mostly ordinary deduction (see LiveScience and U of M). If cars enter a parking lot much faster than other cars exit, you eventually get a nasty traffic jam. You don’t need a model to figure that out.
You do need one if you want to anticipate when a traffic jam will happen, how severe it might be, how long it might last, and the timing of steps that might help reduce or avoid it.
Leaders of cities, counties, states, and nations have to manage large quantities of resources and plan for future outcomes. To do that, they have to make educated guesses about what steps to take now to be ready for what might happen next week, next month, and next year. It’s models that make those guesses educated ones rather than random ones.
Highly technical work performed by exceptionally smart fellow human beings is a gift from God. Christians should recognize that. Because we’ve been blessed with these people and their abilities (and their models) COVID-19 isn’t killing us on anywhere near the scale that the Spanish Flu did in 1918 (Gottlieb is interesting on this). That’s divine mercy!
(Note to those hung up on the topic of “the mainstream media”: none of the sources I linked to here for support are “mainstream media.” Top image: IHME.)
Aaron Blumer 2016 Bio
Aaron Blumer is a Michigan native and graduate of Bob Jones University and Central Baptist Theological Seminary (Plymouth, MN). He and his family live in small-town western Wisconsin, not far from where he pastored for thirteen years. In his full time job, he is content manager for a law-enforcement digital library service. (Views expressed are the author's own and not his employer's, church's, etc.)
- 186 views
… so the saying goes …
You mentioned “highly technical work performed by exceptionally smart fellow human beings … ” - and absolutely no disagreement there, but when there are cases like “climategate” and others, it brings virtually all conclusions drawn by modelers into question. That does not mean we should throw out models entirely, though.
My problem with models is the same problem I have with all of the statistical data - they can be used to present a case however one wants to present it. We have all seen the countless examples of coronavirus statistics and models showing differences of enormous extremes. The majority of us have no idea what the data means, never mind how to properly interpret what is being presented to us.
Before the Age of Internet, modeling was almost exclusively done and used by the experts. That is where it should remain. But it won’t, of course…
Ashamed of Jesus! of that Friend On whom for heaven my hopes depend! It must not be! be this my shame, That I no more revere His name. -Joseph Grigg (1720-1768)
A lot of the issue here is, IMO, that those presenting the models aren’t really presenting them with the confidence ranges—at least I like to think that if people knew the typical confidence range on a model like this, they’d understand that when the model changes—“Man Acts”, as Ludwig von Mises noted—it doesn’t necessarily disprove the model. It simply refines it as we go along.
We might also note that an early model with assumptions that are disproven or modified over time (say as people take steps to prevent infection) is still valuable in that it indicates what could happen if people did not take any actions. It’s like when you tell your child “keep spending like that, you will be bankrupt”—and the fact that your daughter doesn’t get a $5 drink at Starbucks/wherever each day changes your initial assumptions. The point was to modify behavior so the problems didn’t occur, no?
Along these lines, a couple of thoughts from my bike ride yesterday with a pathologist at Mayo; coronavirii tend to mutate quite a bit after a mean time of 3 months, so this one could mutate out of significance soon for us, and second, there’s an open question of whether most of us need to get it to achieve herd immunity, or whether we can reduce infection rates to where only a small minority of people need to get it. I am obviously hoping and praying for the latter.
Aspiring to be a stick in the mud.
“The thing is, to model things accurately, you need reliable data and an accurate understanding of the underlying processes. We have neither of those, so models mostly just generate attractive graphs to conceal the uncertainties.” Glenn Reynolds
Again, source is my pathologist friend, and his discussion with one of the key epidemiologists. Long and short of it is, contra Reynolds, you can get a lot of good data about virulence and lethality (estimates there haven’t changed much), but your overall fatality estimates and rate still depend a lot on von Mises, “Man Acts”. You get a basic set of data assuming people don’t change their behavior (which is, after all, the end point a lot of detractors desire), and that’s the “scared straight” that a lot of people need to avoid certain risky behaviors.
Let’s not confuse mens’ response to the perceived data with total flaws in the models, as too many do here. Anyone who works with these kinds of models, as I have, knows the confidence ranges are wide, and that they’re very sensitive to changes in the assumptions made going in. But again, that doesn’t make them just “attractive graphs to conceal the uncertainties”. They are, rather, representative graphs which illustrate the risks if no action is, or can be, taken.
Aspiring to be a stick in the mud.
A few quick remarks:
- I can’t be an expert on everything.
- I know nothing about modeling, and I won’t even try to research it.
- People who do the modeling are not evil people trying to ruin your life.
- Politicians at local and state level are not evil people trying to ruin your life. This situation is merely a vehicle for displaying their worldviews in a way it hadn’t been displayed before.
- There is no concerted conspiracy by politicians to destroy Christianity. However, there certainly is a worldview that sees no use for Christianity. In that respect, Satan is using COVID-19 to great effect. Wormwood is doing well. Screwtape would be proud.
- Local elections matter.
Tyler is a pastor in Olympia, WA and works in State government.
[Robert Byers]“The thing is, to model things accurately, you need reliable data and an accurate understanding of the underlying processes. We have neither of those, so models mostly just generate attractive graphs to conceal the uncertainties.” Glenn Reynolds
Reynolds is overstating the situation. I was particularly encouraged by the Kaiser Family Foundation piece I linked to in the article. There are actually lots of models being consulted, which is as it should be. When you see different models arriving at basically similar conclusions, your likelihood of making a good call improves quite a bit. It’s like the day both Ted Cruz and AOC started publicly taking COVID-19 seriously… when those two agree on something, it’s pretty sobering—after you get over the shock.
I get that to a lot of people, if your predicted ranges aren’t tight and your probability estimate isn’t like 90%, the thing is “useless,” but I think high level decision makers deal with broad ranges and moderate to low certainty levels all the time. It’s still better than rolling the dice.
(Actually, it might not be, literally… since the probability of rolling a particular number isn’t astronomically low… I thought maybe “consulting an 8 ball” might be a better analogy, but the probability is pretty high on that one. The point is that it’s more responsible to make an imperfectly informed decision than it is to make an uninformed one.)
Views expressed are always my own and not my employer's, my church's, my family's, my neighbors', or my pets'. The house plants have authorized me to speak for them, however, and they always agree with me.
Here is the problem. Let’s say I am modeling a particle physics experiment, or tomorrow’s weather. No problems there. It is pure science mixed with good prediction techniques. No bias there. The thing is, when you get to other topics, there can be, and likely are, agendas mixed in with the model.
Say I am a drug company and I have spent millions making a drug for dogs. Yes, what I am about to say has happened, I am not making it up. I have first hand info on it. Say you are a drug maker for dogs. You have this great new drug you have been working on and the problem is, the clinical trial just isn’t working out. The drug does not work… but you try to massage, shall we say, the statisticians to write the report in such a way as the drug does work. Yep, happened. And it happens.
Same thing with “models.” Want a rosey economic forecast? I can provide it. Want a dire one? Same price. Climate change… hey there is a lot of money there. Scientist have no greed whatsoever, right… Worse, a lot of scientists, and I know a lot of them, are flat out socialists and/or communists. Yep. Communists. They are LOVING the fact that the economy is shut down right now. Yep. That’s a fact, not an assumption.
So, when it comes to models, you have to look at them with a discriminatory eye. Is this real? Reasonable?
I think the epidemiology community came at Trump with the 2 million + death numbers and even he, the immoral scum bag that many here claim he is… even he gasped at that potential and acted on the models.
It now seems the model were wrong. Not just a little… a lot. Why? Well, I’d only guess at an answer. Let me paraphrase John E from today on another topic, isn’t this an election year?
Maranatha!
Don Johnson
Jer 33.3
@Mark: your narrative is off on almost every point. (a) It’s very hard to put bias into a model. That normally happens with the output, and mostly happens in the popular press/media; (b) there is no qualitative difference between weather modeling and disease transmissibility modeling or hospitcal capacity modeling; (c) there is no one model behind the flattening the curve strategy—there is no “the model” to be “wrong”; (d) the models have not been found to be “wrong.”
@Don
“JASON RICHWINE is a public-policy analyst and a contributor to National Review Online.”
It’s significant that Jason, the author of that piece, isn’t an epidemiologist or virologist or has any background in statistical analysis.
I haven’t had chance to dig into much, but he doesn’t seem to be reading Professor Gonsalves fairly…. the piece sounds like typical populist resentment of the idea that some people have studied and labored in their field long and hard enough to have truly superior skill. … why anybody should resent that is a long story, but it’s foolish.
I’m not saying the experts are always right and should never be questioned. We shouldn’t expect them to have infinite patience with people who demand explanations but don’t really have the background to understand an explanation… or to have the skill to teach them what they’d have to know in order to understand.
When the plumber is doing stuff in my basement I don’t stand by and demand to understand why he’s doing everything he’s doing. It’s usually better to let him do his work and then try to use it wisely when he’s done.
Views expressed are always my own and not my employer's, my church's, my family's, my neighbors', or my pets'. The house plants have authorized me to speak for them, however, and they always agree with me.
when it does not match what happened. Now, the reason for that may be that you changed one of the factors. In COVID, maybe social distancing took us from 2.2 million deaths to the 60,000 now projected…. or maybe, just maybe, experts floated the 2.2 million to get the result they wanted.
The point is people sometimes use models to get the answer they are looking for.
If we’re going to talk particle/quantum physics, the comments of Mickelson at the opening of the Ryerson lab at the U. of Chicago come to mind—the claim that future discoveries would be in the 5th and 6th decimal place. A young patent inspector (Einstein) put the kibosh on that just a few years later, and it’s worth noting that there was tremendous debate over the origins and nature of quantum physics—it wasn’t just the Nazis that derided it as “Jewish” physics. So let’s not pretend that bias is exclusively an issue here, or in climatology. Read also about the debates and antics in the Royal Society at the time of Newton.
But that said, what’s going on here is emphatically NOT bias, and it is emphatically NOT that “the models are wrong”. Rather, it is that Ro depends strongly on human responses to a crisis, and hence it drops precipitously when (for example) people stop riding the subway—that’s when things started to turn around in New York City, for example, among other factors.
There is room for discussion about which “shelter in place” orders deliver meaningful reduction in infection rates, but please, let’s not discard thousands of years of knowledge about epidemics by claiming that since human behavior has changed, that there is no geometric/exponential progression of disease. Again, it’s von Mises in “Human Action”; “Man Acts.”
Really, if you argue that “the model is wrong” in this case, you can by the very same logic say that the models for cholera were wrong in 1854 when shutting down a well pump in London stopped an outbreak cold. No, the models worked, but human behavior changed, and we are all the better for it.
Aspiring to be a stick in the mud.
I keep seeing this “Man Acts” argument trotted out in defense of the lousy IHME model that has been the basis for many government decisions. Per the CDC website:
“This model assumes social distancing stays in place until the pandemic, in its current phase, reaches the point when COVID-19 deaths are less than 0.3 per million people. Based on these latest projections, IHME expects social distancing measures to be in place through the end of May.”
Here’s the thing. That’s the SAME assumption they used when they projected 200,000 deaths. They’ve now dropped their projection to 60,000. If they’re telling the truth about their own model, the change CANNOT be the result of man acting or social distancing being implemented. They said they already assumed it would be implemented in arriving at those numbers. Dr Birx used this figure in a White House briefing when she said of social distancing “if we do things almost perfectly,” the death toll would be up to 200,000.
The concept of modeling is not junk. This particular model is junk. And that’s not (just) some kind of right wing talking point. From the Becker Hospital Review on Friday (4/17): “COVID-19 projections from the University of Washington’s Institute for Health Metrics and Evaluation in Seattle are unreliable and should not be used to inform national policy, epidemiologists told STAT. ‘It’s not a model that most of us in the infectious disease epidemiology field think is well suited’ for projecting COVID-19 deaths, Marc Lipsitch, PhD, an epidemiologist at the Harvard T.H. Chan School of Public Health in Boston, told the publication.”
Not just Christians but all thinking people should dismiss the IHME model with extreme prejudice.
The models that governments around the world followed in creating this crisis are so off in their projections as to be laughable. When the non-expert can easily see they are so far off, the thinking person shouldn’t defend the so-called expert projections.
What is wrong with the models? They started with insufficient data and made wild predictions. Past history should have made them more cautious, but it didn’t.
As a friend of mine said this morning, “We’re going to be paying for this one for a long time.”
I said, “No, our grandchildren will…”
Maranatha!
Don Johnson
Jer 33.3
[Robert Byers]The concept of modeling is not junk. This particular model is junk.
Yes
[Bert Perry]If we’re going to talk particle/quantum physics, the comments of Mickelson at the opening of the Ryerson lab at the U. of Chicago come to mind—the claim that future discoveries would be in the 5th and 6th decimal place. A young patent inspector (Einstein) put the kibosh on that just a few years later, and it’s worth noting that there was tremendous debate over the origins and nature of quantum physics—it wasn’t just the Nazis that derided it as “Jewish” physics. So let’s not pretend that bias is exclusively an issue here, or in climatology. Read also about the debates and antics in the Royal Society at the time of Newton.
True, but not the point Bert. For the record, when I referred to particle physics I was thinking more like models predicting the mass of a Higgs Boson… that is pure science. Weather forecasting is pure science.
When you enter into hurricane forecasting… now science gets a little edgier because people are trying to get others to act. Any time you use models to make decisions, bias can and does enter in. People have preferences and pick the projection they like, or favor, or want to make happen, or want to impose. Whatever.
Discussion