Christians Shouldn’t Be Dismissive of Scientific Modeling
Image
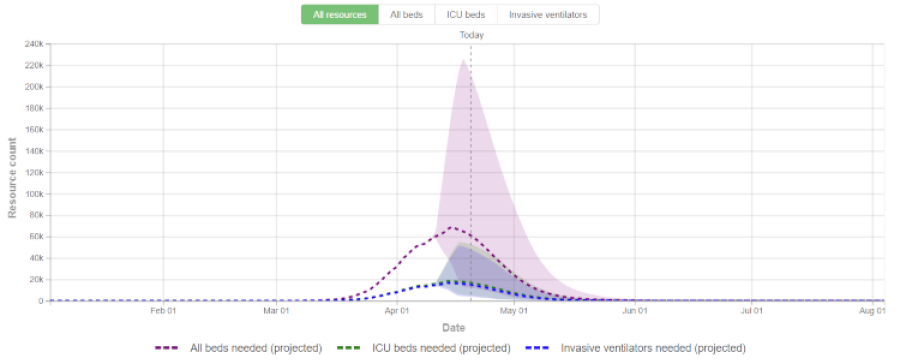
Projections from an IHME model.
Over the last several weeks I’ve encountered a range of negative views toward the models epidemiologists have been using in the struggle against COVID-19. Skepticism is a healthy thing. But rejecting models entirely isn’t skepticism. Latching onto fringe theories isn’t skepticism. Rejecting the flattening-the-curve strategy because it’s allegedly model-based isn’t skepticism either.
These responses are mostly misunderstandings of what models are and of how flattening-the-curve came to be.
I’m not claiming expertise in scientific modeling. Most of this is high school level science class stuff. But for a lot of us, high school science was a long time ago, or wasn’t very good—or we weren’t paying attention.
What do models really do?
Those tasked with explaining science to us non-scientists define and classify scientific models in a variety of ways.
The Stanford Encyclopedia of Philosophy, for example, describes at least 8 varieties of models, along with a good bit of historical and philosophical background. They’ve got about 18,000 words on it.
A much simpler summary comes from the Science Learning Hub, a Science-education project in New Zealand. Helpfully, SLH doesn’t assume readers have a lot of background.
In science, a model is a representation of an idea, an object or even a process or a system that is used to describe and explain phenomena that cannot be experienced directly. Models are central to what scientists do, both in their research as well as when communicating their explanations. (Scientific Modeling)
Noteworthy here: models are primarily descriptive, not predictive. Prediction based on a model is estimating how an observed pattern probably extends into what has not been observed, whether past, present, or future.
Encyclopedia Britannica classifies models as physical, conceptual, or mathematical. It’s the mathematical models that tend to stir up the most distrust and controversy, partly because the math is way beyond most of us. We don’t know what a “parametrized Gaussian error function” is (health service utilization forecasting team, p.4; see also Gaussian, Error and Complementary Error function).
But Christians should be the last people to categorically dismiss models. Any high school science teacher trained in a Christian university can tell you why. I’ve been reminded why most recently in books by Nancy Pearcy, Alvin Plantinga, William Lane Craig, and Samuel Gregg: Whether scientists acknowledge it or not, the work of science is only possible at all because God created an orderly world in which phenomena occur according to patterns in predictable ways. For Christians, scientific study—including the use of models to better understand the created order—is study of the glory of God through what He has made (Psalm 19:1).
Most of us aren’t scientists, but that’s no excuse for scoffing at one of the best tools we have for grasping the orderliness of creation.
Should we wreck our economy based on models?
The “models vs. the economy” take on our current situation doesn’t fit reality very well. Truth? The economy is also managed using models. A few examples:
- Calculating the unemployment rate
- Unemployment forecasting (also this)
- Business forecasting
- Cost Modeling
Beyond economics, modeling is used all the time for everything from air traffic predictions to vehicle fire research, to predictive policing (no, it isn’t like “Minority Report”).
Models are used extensively in all sorts of engineering. We probably don’t even get dressed in the morning without using products that are partly the result of modeling—even predictive modeling—in the design process.
Christians should view models as tools used by countless professionals—many of whom are believers—in order to try to make life better for people. Pastors have books and word processors. Plumbers have propane torches. Engineers and scientists have models. They’re all trying to help people and fulfill their vocations.
(An excellent use of predictive mathematical modeling…)
Why are models often “wrong”?
An aphorism about firearms says, “Guns don’t kill people; people kill people.” Implications aside, it’s a true statement. It’s also true that math is never wrong; people are wrong. Why? Math is just an aspect of reality. In response to mathematical reality, humans can misunderstand, miscalculate, and misuse, but reality continues to be what it is, regardless.
The fact that the area of a circle is always its radius squared times an irrational (unending) number we call “pi” (π) remains true, no matter how many times I misremember the formula, plug the wrong value in for π, botch the multiplication, or incorrectly measure the radius.
The point is that models, as complex representations of how variables relate to each other and to constants, are just math. In that sense, models are also never “wrong”—just badly executed or badly used by humans. That said, a model is usually developed for a particular purpose and can be useless or misleading for the intended purpose, so, in that sense, “wrong.”
When it comes to using models to find patterns and predict future events, much of the trouble comes from unrealistic expectations. It helps to keep these points in mind:
- Using models involves inductive reasoning: data from many individual observations is used in an effort to generalize.
- Inductive reasoning always results in probability, never certainty.
- The more data a model is fed, and the higher the quality of that data, the more probable its projections will be.
- When data is missing for parts of the model, assumptions have to be made.
- Changes in a model’s predictions are not really evidence of “failure.” As the quantity and quality of data changes, and assumptions are replaced with facts, good models change their predictions.
- True professionals, whether scientists or other kinds of analysts, know that models of complex data are only best guesses—and they don’t claim otherwise.
- The professionals that develop and use models in research are far more tentative and restrained in their conclusions than people who popularize the findings (e.g., the media).
In the case of COVID-19, one of the most influential models has been one of IHME’s (Institute for Health Metrics and Evaluation). Regarding that model, an excellent Kaiser Family Foundation article notes:
Models often present “best guess” or median forecasts/projections, along with a range of uncertainty. Sometimes, these uncertainty ranges can be very large. Looking at the IHME model again, on April 13, the model projected that there would be a 1,648 deaths from COVID-19 in the U.S. on April 20, but that the number of deaths could range from 362 to 4,989.
Poor design and misuse have done some damage to modeling’s reputation. Some famous global-warming scandals come to mind. But in the “Climategate” controversy, for example, raw data itself was apparently falsified. The infamous hockey stick graph appears to have involved both manipulated raw data and misrepresentation of what the model showed. Modeling itself was not the problem.
(XKD isn’t completely wrong … there is such a thing as “better garbage”)
Why bother with models?
Given the uncertainty built into predictive mathematical models, why bother to use them? Usually, the answer is “because we don’t have anything better.” Models are about providing decision-makers, who don’t have the luxury of waiting for certainty, with evidence so they don’t have to rely completely on gut instinct. It’s not evidence that stands alone. It’s not incontrovertible evidence. It’s an effort to use real-world data to detect patterns and anticipate what might happen next.
As for COVID-19, the idea that too many sick at once would overwhelm hospitals and ICUs, and that distancing can help slow the infection rate and avoid that disaster, isn’t a matter of inductive-reasoning from advanced statistical models. It’s mostly ordinary deduction (see LiveScience and U of M). If cars enter a parking lot much faster than other cars exit, you eventually get a nasty traffic jam. You don’t need a model to figure that out.
You do need one if you want to anticipate when a traffic jam will happen, how severe it might be, how long it might last, and the timing of steps that might help reduce or avoid it.
Leaders of cities, counties, states, and nations have to manage large quantities of resources and plan for future outcomes. To do that, they have to make educated guesses about what steps to take now to be ready for what might happen next week, next month, and next year. It’s models that make those guesses educated ones rather than random ones.
Highly technical work performed by exceptionally smart fellow human beings is a gift from God. Christians should recognize that. Because we’ve been blessed with these people and their abilities (and their models) COVID-19 isn’t killing us on anywhere near the scale that the Spanish Flu did in 1918 (Gottlieb is interesting on this). That’s divine mercy!
(Note to those hung up on the topic of “the mainstream media”: none of the sources I linked to here for support are “mainstream media.” Top image: IHME.)
Aaron Blumer 2016 Bio
Aaron Blumer is a Michigan native and graduate of Bob Jones University and Central Baptist Theological Seminary (Plymouth, MN). He and his family live in small-town western Wisconsin, not far from where he pastored for thirteen years. In his full time job, he is content manager for a law-enforcement digital library service. (Views expressed are the author's own and not his employer's, church's, etc.)
- 186 views
For those who value reading primary sources, IHME has an update as of yesterday.
I’d personally lilke to see more history on how the models (note the plural… because there really isn’t even a single IHME model) they’re using now came to be. In particular, what components of it are shared with models used in past outbreaks.
Anyway, here’s IHME’s 4/22 Update
An interesting side note. Lots of model critics I heard from argued that the models were wrong because they were government projects to control us. Turns out IHME is mostly funded by Bill Gates’ foundation… private enterprise, my friends. So, quick, pivot! Now the model is wrong because Bill Gates is “definitely up to something.”
The commitment we’re seeing is to a “this has has to be a huge mistake/sinister plot” narrative rather than to actually understanding the situation.
Views expressed are always my own and not my employer's, my church's, my family's, my neighbors', or my pets'. The house plants have authorized me to speak for them, however, and they always agree with me.
Interesting bit in IHME’s update on counting confrimed COVID deaths vs. counting presumed COVID deaths. (From the linked page above)
New York. As mentioned yesterday, about midway through the COVID-19 epidemic in New York City (NYC), reporting of confirmed and presumed deaths started to occur separately. To account for this important distinction, we have instituted an alternative data processing step for NYC and thus New York state. To better track with the time series of confirmed cases from the NYC Department of Health and Mental Hygiene (DOHMH), we now use the NYC data captured by the NY Times GitHub repository.
To account for presumed deaths, we use the most recent day of reporting and now take the difference between NYC DOHMH total COVID-19 deaths (the sum of presumed and confirmed deaths from the virus) and subtract reported deaths for that day based on the NY Times dataset. We then redistribute the remainder of deaths proportionate to the daily COVID-19 deaths from the NY Times data source.
A few things to note here:
1. On the question of whether to count people who died w/COVID like symptoms vs. those who died who were known to have COVID… They’re quite aware of that distinction (this does not surprise me at all) and want to handle that data as accurately as possible. It’s in their best interest to be as accurate as possible in counting deaths because projections are less accurate if the data going in is less accurate.
2. IHME is completely transparent about where its data comes from. … not quite as transparent about what calculations they do—at least in this report. But read that page and tell honesty that this has a “bad people trying to accomplish a political agenda” feel to it. Seriously.
3. The assumption that these folks lack common sense just because they’re specialists is … not consistent with common sense. Maybe we just don’t personally know enough really smart people. I’d like to know more, but those I do know are, to a man or woman, in possession of as much common sense as everyone else. On the whole, a bit more.
Politicians, on the other hand… not so much. But leaders of cities, counties, states, and nations have very difficult work in normal circumstances. It’s certainly extra difficult right now to figure out what to do. Putting everybody in their state out of work is not a recipe for re-election. These leaders would not sacrifice their own careers in order to “defeat Trump.” That angle on things doesn’t fit “common sense” at all.
Governors have no reason I can think of to want to cripple their economies other than the belief that they don’t have any choice.
So suggestions to those who gravitate toward a more hostile view of things…
- Think local rather than national and you’ll make better sense of things. Local politicians aren’t as interested in defeating or electing U.S. Presidents as they are in keeping their own power.
- Just as a thought experiment, try starting out with the assumption that the people involved in these efforts are more like you than not. Imagine they’re humans with families, and homes, and training, and jobs, and about the average amount of good sense and good will.
- This is not only a very likely assumption, but it’s kind of fundamental to Christian charity… but in any case, it doesn’t make sense to assume malice and/or stupidity without evidence. If we assume it first, it’s easy to cherry pick evidence to confirm. If we start out giving people a little respect, then we’re more likely to look at evidence fairly and believe something closer to truth.
Views expressed are always my own and not my employer's, my church's, my family's, my neighbors', or my pets'. The house plants have authorized me to speak for them, however, and they always agree with me.
In New York State, with officially 263k confirmed cases (~1.4%), antibody testing of 3000 people suggested about a 13.9% infection rate among those “out and about”. Those in their homes were not part of the study, so it’s probably not as good as “10:1 rate of infection to symptoms”, but it’s very hopeful to suggest a fair amount of unknown immunity out there.
The flip side; a good portion of those people were probably passing COVID-19 around without knowing what they were doing. This is a big part of the broad social restrictions; until you’ve got a good notion of who can pass it on, it’s going to be hard to do a targeted quarantine. It will also be difficult given that the virus apparently already has 27 or so different known mutations—at a certain point, those are going to go far enough that current tests won’t work. Again, these virii mutate quickly.
Aspiring to be a stick in the mud.
[Bert Perry]Again, these virii mutate quickly.
Not necessarily. I’ve read a few sources that have said SARS-CoV-2 actually doesn’t mutate as much as other coronavirii; as with all, yes, it has and will mutate, but the mutations are much slower and less significant than with previous ones.
But regarding New York and antibodies, that’s really what needs to happen - herd immunity. But, how to get there is the challenge - that balancing act that the experts and politicians are trying to find. The fastest way out of COVID-19 is a high infection rate and associated large quantities of death.
Ashamed of Jesus! of that Friend On whom for heaven my hopes depend! It must not be! be this my shame, That I no more revere His name. -Joseph Grigg (1720-1768)
COVID-19 may not mutate like other coronavirii, but with 27 variants (probably more now) already, I think it’s safe to say it’s a fairly rapidly moving target. To draw a picture, one hypothesis about why H1N1 went out is that it mutated out of significance.
And nothing against herd immunity, but given that the process of obtaining that herd immunity is rather rough on a lot of people, I’m still holding out hope that what we’re supposed to be doing will slow things down enough so either the virus will mutate into insignificance, or the last few people with it get healthy and it just dies out. Unfortunately, I don’t know that you can model how quickly errors in a virus accumulate to render it uninteresting from the ICU nurse’s point of view. I’m guessing that anyone who developed a fairly adaptable/portable model of this would be able to count on a call from Oslo when the Nobels were announced.
Aspiring to be a stick in the mud.
Got curious about the alleged prediction from “the model” that 2.2 million would die of COVID-19 in the US.
What actually happened…
- scientists in UK used the UK’s Imperial Model
- They wrote a report full of numbers describing worst case scenarios in various regions
- The worst case scenario was that people would not change they’re behavior at all in response to the spread of the virus
- This hypothetical absolute status quo is silly, but it was clearly communicated in the report as the scenario being described
- One of the regions was US, and the absolute status quo outcome they estimated was 2.2 million deaths
This write up at Reason seems to be very well documented: https://www.google.com/amp/s/reason.com/2020/03/31/2-2-million-american…
From the report
In the (unlikely) absence of any control measures or spontaneous changes in individual behaviour, we would expect a peak in mortality (daily deaths) to occur after approximately 3 months (Figure 1A). In such scenarios, given an estimated R0 of 2.4, we predict 81% of the GB and US populations would be infected over the course of the epidemic. Epidemic timings are approximate given the limitations of surveillance data in both countries: The epidemic is predicted to be broader in the US than in GB and to peak slightly later. This is due to the larger geographic scale of the US, resulting in more distinct localised epidemics across states (Figure 1B) than seen across GB. The higher peak in mortality in GB 16 March 2020 Imperial College COVID-19 Response Team is due to the smaller size of the country and its older population compared with the US. In total, in an unmitigated epidemic, we would predict approximately 510,000 deaths in GB and 2.2 million in the US, not accounting for the potential negative effects of health systems being overwhelmed on mortality.
The report even says this outcome was “unlikely.”
So… there never was any model that predicted 2.2 million in the US would die.
There was, in fact, a model that predicted that 2.2 million were “unlikely” to die.
As for the observation that this many might die if there was no response whatsoever… for all we know, that number might be correct.
The problem was, apparently, that the Imperial Model wasn’t designed to factor in how people and governments actually behave.
Maybe this was done in order to reduce the number of variables and increase the probability of a narrower range of projected deaths?
No idea.
The Reason piece goes on to point out
Unfortunately, the media generally failed to make clear that this was not a real-world projection, and were abetted in that malfeasance by the lead author of the study, Neil Ferguson. For example, Dr. Ferguson told the New York Times on March 16th that the potential health impacts were comparable to the devastating 1918 influenza outbreak. That outbreak killed approximately .6% of the U.S. population, which today would amount to around two million people, or very close to the fanciful 2.2 million projection. Nor does Ferguson seem to have made any effort to correct Kristof et al. when they wrongly claimed that 2.2 million was a realistic worst-case scenario.
And the media continues to misreport what the study said.
So, I don’t know what all this proves, but it doesn’t prove the models are bad. It seems pretty in line with what I wrote in my article: math is never wrong; people are.
Views expressed are always my own and not my employer's, my church's, my family's, my neighbors', or my pets'. The house plants have authorized me to speak for them, however, and they always agree with me.
Earlier I cited an article that gave third party reporting to something Dr. Fauci may or may not have said.
The following quote attributes Dr. Fauci directly:
“Whenever the models come in, they give a worst-case scenario and a best-case scenario. Generally, the reality is somewhere in the middle. I’ve never seen a model of the diseases that I’ve dealt with where the worst case actually came out. They always overshoot,” Dr. Anthony Fauci, a key member of the White House’s coronavirus task force, told CNN’s Jake Tapper on “State of the Union.”
Source? CNN, March 30, 2020 - emphasis mine
So we should believe modeling exactly why?
Maranatha!
Don Johnson
Jer 33.3
[Don Johnson]Earlier I cited an article that gave third party reporting to something Dr. Fauci may or may not have said.
The following quote attributes Dr. Fauci directly:
“Whenever the models come in, they give a worst-case scenario and a best-case scenario. Generally, the reality is somewhere in the middle. I’ve never seen a model of the diseases that I’ve dealt with where the worst case actually came out. They always overshoot,” Dr. Anthony Fauci, a key member of the White House’s coronavirus task force, told CNN’s Jake Tapper on “State of the Union.”
Source? CNN, March 30, 2020 - emphasis mine
So we should believe modeling exactly why?
Perhaps….because Dr. Fauci does? Perhaps because he understands the role of variance and human action in where the models go, and he’s still staking his career and life (the man is 79, after all, prime COVID vulnerability age) on the response to this epidemic? Perhaps because he, and the agency he’s headed since 1984, has been calculating estimates of virulence and lethality accepted by medical experts around the world, and whose models have been used to address pandemics (ebola, H1N1, avian flu, etc..) around the world?
Honestly, Don, I don’t think you’d take it well if you were treated with the contempt you’re pouring out on many of the top epidemiologists of the world. Maybe extend some of the same courtesy you’d expect, and maybe even consider learning about WHY Dr. Fauci isn’t particularly bothered that early estimates are higher than the actual result?
(hint; it has something to do with statistical confidence ranges and the fact that people sometimes respond to perceived threats.. )
Aspiring to be a stick in the mud.
Have you been alive the last 3.5 years when the media uses things like this to manipulate news stories to make Trump look like the worst president ever? Or have you been stuck under a rock?
[Mark_Smith]Have you been alive the last 3.5 years when the media uses things like this to manipulate news stories to make Trump look like the worst president ever? Or have you been stuck under a rock?
Mark, if this is a conspiracy where huge numbers of doctors are faking death certificates to make it look like someone with no symptoms of COVID died of it, don’t you think that someone would be raising H*** about the matter? Sixty thousand corpses/funerals and hundreds of thousands of hospitalizations are pretty darned hard to fake, don’t you think?
It’s possible to maintain a conspiracy when you’ve got only a few dozen people holding all the cards (e.g. the Mueller investigation), and the relevant data are largely hidden from the public—and keep in mind there that as the data are coming out, all H*** is breaking loose. It’s also halfway possible to maintain a false narrative when one side of the argument is funding all the research—e.g. global warming. In this case, however, you’ve got millions of witnesses to how their loved ones died after showing the symptoms of COVID.
No doubt that the media have the long knives out for Trump, but the data I’m working on are pretty darned hard to fake.
Aspiring to be a stick in the mud.
You still don’t get it. Have 58000+ people died of COVID in the US. Yes! Personally, I would consider 70K deaths by August to be a tremendous success in pandemic terms. Was the US unprepared for this? Absolutely. ANY PRESIDENT would have been unprepared because people do not like spending money preparing for things… they want low taxes, not stockpiles of PPE and ventilators. They want cheap stuff, not PPE made in Michigan. So have it made in China… no problem right? Every president and every Congress has known this was going to eventually happen and NO ONE did anything about it. Not just Trump.
You act like I’m some conspiracy nut. Far from it.
What I am talking about is how every news outlet uses the models to say we need “better leadership.” Let’s write 5 articles today on how Trump didn’t prepare well enough for this virus. Things like that. The sole reason they use models is to attack Republicans. That is what I despise. That is what makes the models pointless.
Of course models “predict the worse case and the best case.” That is not the point.
The point is how models are being used to weaken (or perhaps destroy) a presidency and a nation just for the political, social, and cultural benefit of one side who controls the main stream media.
And finally Bert, please stop acting like you are modeling this epidemic. Its creepy. (“but the data I’m working on are pretty darned hard to fake.”)
Mark, I’m going to have to suggest that it’s you who doesn’t get it. No, the reason journalists use models is not solely to attack the President, but because they happen to be news. Moreover, the solution to the problem of journalists misunderstanding models is emphatically not for us to attack the models. The proper response is to explain them in their proper context.
And yes, I do happen to be doing a simple model to try and clarify what’s going on. This is something that anyone who remembers high school health class and 1st semester calculus ought to be able to do, and I make no apologies for it. It is the kind of thing that we all ought to be doing to start shedding light on the subject instead of merely heat and anger. Give it a try.
Aspiring to be a stick in the mud.
is the journalists are biased political operatives. Its not “lack of understanding” about the context of the model.
[Bert Perry]And yes, I do happen to be doing a simple model to try and clarify what’s going on. This is something that anyone who remembers high school health class and 1st semester calculus ought to be able to do, and I make no apologies for it. It is the kind of thing that we all ought to be doing to start shedding light on the subject instead of merely heat and anger. Give it a try.
Bert, if you want to do that, go ahead. But stop pretending you are a public health modeler. You aren’t.
“Whenever the models come in, they give a worst-case scenario and a best-case scenario. Generally, the reality is somewhere in the middle. I’ve never seen a model of the diseases that I’ve dealt with where the worst case actually came out. They always overshoot,” Dr. Anthony Fauci, a key member of the White House’s coronavirus task force, told CNN’s Jake Tapper on “State of the Union.”
This is consistent with what I’ve been saying and said in the article. I explain there both why models work this way and why they’re still useful.
It’s really not hard to understand that a range of possible outcomes with a range of probability is more useful than no information at all. … I have to think that folks not getting this are just not trying very hard.
Nothing I can do about that.
@Mark: “The media” do not control the models. They don’t develop them, they don’t collect the data for them, they don’t fund them, they sometimes try to interpret them—but usually leave even that to others… then interpret the interpretation.
So, the “debate” kind of goes like this:
- Aaron: various facts and reasoning from them (analysis, and opinion downstream of that)
- Response: hey, look over there at that awful stuff!!!!
Well, that’s one way to interact with information. It’s not a truth-loving way, though. And as Christians we’re called to love truth and grow in truth-loving habits. Every single one of us.
Views expressed are always my own and not my employer's, my church's, my family's, my neighbors', or my pets'. The house plants have authorized me to speak for them, however, and they always agree with me.
Discussion